1 Foundational Technology Layers
1.3
Embedded Software and Beyond
The Artemis/Advancy report1 states that "the investments in software technologies should be on at least an equal footing with hardware technologies, considering the expected growth at the higher level of the value chain (Systems of Systems, applications and solutions)". According to the same report, embedded software and software engineering tools are part of the six technology domains needed for embedded intelligence. Embedded intelligence means incorporating AI algorithms (“classic” or ML ones) in devices or components to give them the ability to reflect on their own state (e.g. operational performance, usage load, environment), execute tasks independently, adjust to novel circumstances, and make data-driven decisions without human input. Such devices will operate in a robust and resilient way, e.g. independent of internet connectivity and are the necessary step towards the next level of digitalisation and sustainability. In this context, embedded intelligence supports the green deal initiative, as one of the tools for enhancing sustainability.
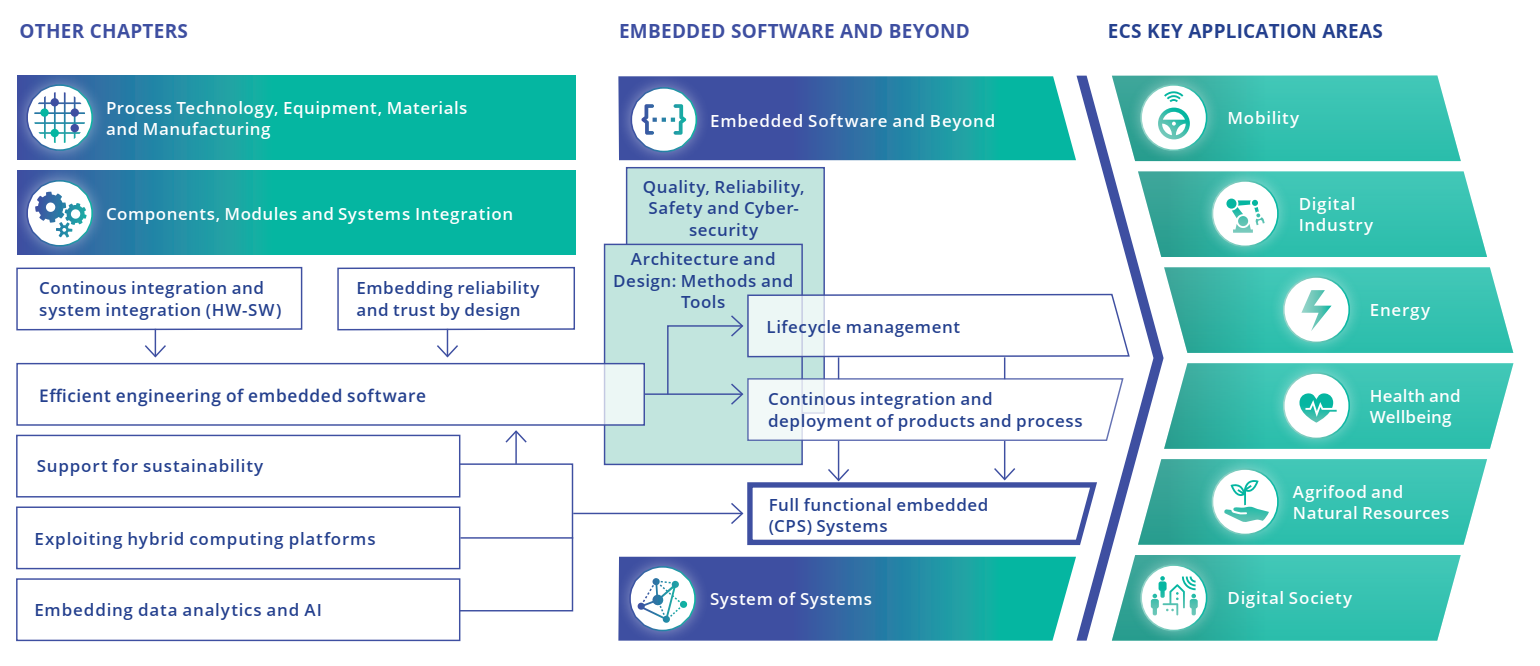
Figure 1.3.1 illustrates the role and positioning of the Embedded Software and Beyond Chapter in the ECS- SRIA. The Chapter on Components Modules and System Integration focuses on functional hardware components and systems that compose the embedded and cyber-physical systems (CPS), considered in this Chapter. While the System of Systems (SoS) Chapter is based on independent, fully functional systems, products and services (which are also discussed in this Chapter), they are also the constituents of SoS-based solutions. The Architecture and Design: Methods and Tools Chapter examines engineering processes, methods, and tools, while this Chapter focuses more on the technology stack of Embedded Software and Beyond. For the discussion on safe, trustworthy, and explainable AI in the context of embedded intelligence, this Chapter is also linked to Quality, Reliability, Safety and Cybersecurity (Chapter 2.4).
This Chapter is called Embedded Software and Beyond to stress that embedded software is more than “just software”: it is a key component of any system’s embedded intelligence, it enables systems to act on external events, and it enables inter-system communication.
Most importantly, Embedded software empowers Embedded and cyber-physical systems (ECPS) to play a key role in solutions for digitalisation in almost every application domain (cf. Chapters 3.1-3.6). From a functional perspective, the role of Embedded Software is becoming increasingly dominant because of the new software-enabled functionalities ECPS (e.g. cars, trains, airplanes and health equipment) need to provide (including aspects as security, privacy and autonomy). In these systems, most of the innovation comes from software, nowadays. ECPS also form the backbone of SoS (e.g. smart cities, air traffic management), providing required interconnection and interoperability. Owing to all these factors, ECPS are an irreplaceable part of the strive towards digitalisation of our society.
At the same time, ECPS need to exhibit required quality properties (e.g. safety, security, reliability, dependability, sustainability, and, ultimately, trustworthiness). Furthermore, due to their close integration with the physical world, ECPS must consider the dynamic and evolving aspects of their environment to provide deterministic, high-performance, and low-power computing, especially when processing intelligent algorithms. Increasingly, software applications will run as services on distributed SoS involving heterogeneous devices (e.g: servers, edge devices) and networks, with a diversity of resource restrictions. In addition, it is required from ECPS that their functionalities and hardware capabilities evolve and adapt during their lifecycles – e.g. through updates of software or hardware in the field and/or by learning. Building these systems and guaranteeing their previously mentioned quality properties, along with supporting their long lifetime and certification, requires innovative technologies in the areas of modelling, software engineering, model-based design, verification and validation (V&V) technologies, and virtual engineering. These advances need to enable engineering of high-quality, certifiable ECPS that can be produced (cost-)effectively (cf. Chapter 2.3, Architecture and Design: Methods and Tools).
Common challenges in embedded software and its engineering for ECPS include:
-
Interoperability.
-
Complexity of requirements and code (safety, security, performance).
-
Quality (dependability, sustainability, performance, trustworthiness).
-
Lifecycle (maintainability, extendibility).
-
Efficiency, effectiveness, and sustainability of software development.
-
Adaptability to, and the dynamic environment of ECPS.
-
Maintenance, integration, rejuvenation of legacy software solutions.
To enable ECPS functionalities and their required level of interoperability, the engineering process will be progressively automated and will need to be integrated in advanced SoS engineering covering the whole product during its lifetime. Besides enabling new functionalities and their interoperability, it will need to cover non-functional requirements (safety, security, run-time performance, reliability, dependability, sustainability, and, ultimately, trustworthiness) visible to end users of ECPS, and to also satisfy quality requirements important to engineers of the systems (e.g. evolution, maintenance). This requires innovative technologies that can be adapted to the specific requirements of ECPS and, subsequently, SoS.
Further complexity will be imposed by the introduction of Artificial Intelligence (AI), machine-to-machine (M2M) interaction, new business models, and monetisation at the edge. This provides opportunities for enhancing new engineering techniques like AI for SW engineering, and SW engineering for AI. Future software solutions in ECPS will solely depend on new software engineering tools and engineering processes (e.g. quality assurance, Verification and Validation (V&V) techniques and methods on all levels of individual IoT and in the SoS domain).
Producing industrial software, and embedded software in particular, is not merely a matter of writing code: to be of sufficient quality, it also requires a strong scientific foundation to assure correct behaviour under all circumstances. Modern software used in products such as cars, airplanes, robots, banks, healthcare systems, and the public services comprises millions of lines of code. To produce this type of software, many challenges have to be overcome. Even though software in ECPS impacts everyone everywhere, the effort required to make it reliable, maintainable and usable for longer periods is routinely underestimated. As a result, every day there are news articles about expensive software bugs and over-budget or failed software development projects. Also, big challenges with correctness and quality properties of software exist, as human well-being, economic prosperity, and the environment depend on it. There is a need to guarantee that software is maintainable and usable for decades to come, and there is a need to construct it efficiently, effectively and sustainably. Difficulties further increase when legacy systems are considered: information and communications technology (ICT) systems contain crucial legacy components at least 30 years old, which makes maintenance difficult, expensive, and sometimes even impossible.
The scope of this Chapter is research that facilitates engineering of embedded software used for ECPS, enabling digitalisation through the feasible and economically accountable building of SoS with necessary quality. It considers:
-
Challenges that arise as new applications of ECPS emerge.
-
Continuous integration, delivery and deployment of products and processes.
-
Engineering and management of ECPS during their entire lifecycle, including sustainability requirements.
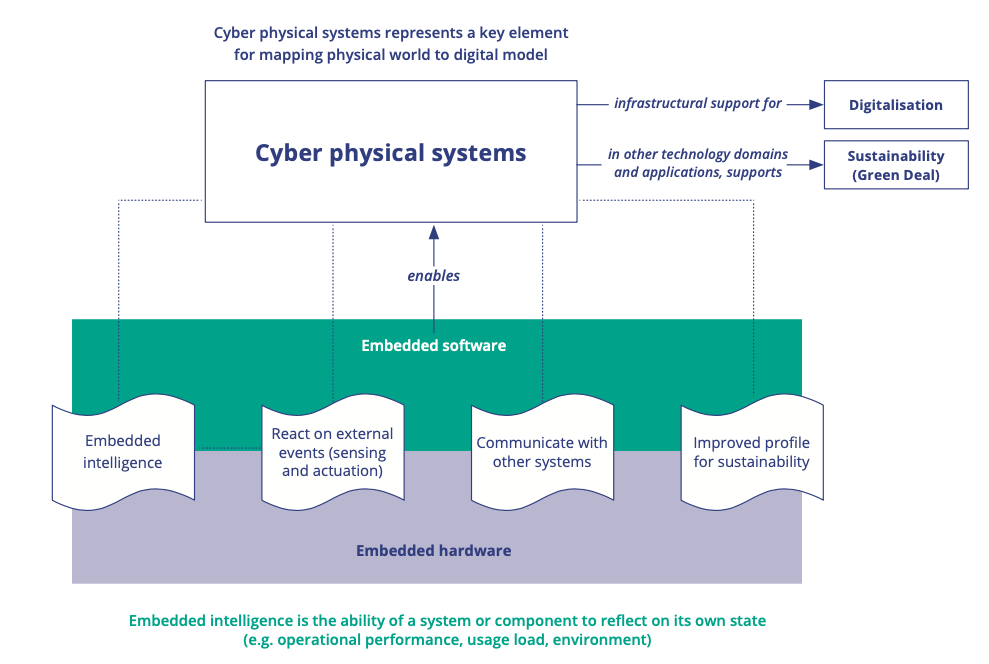
Quantum Technologies
Quantum technology has drawn a growing amount of attention in recent years. This short text briefly explains the three main topics of this fields. An inventory is made of the impact of quantum technologies on embedded software and beyond.
The Three Main Topics of Quantum Technology
Quantum computing, quantum internet and quantum sensing are the three main topics of quantum technology. Let’s take a look at all three.
Quantum Computing Quantum computing is the most captivating of the three. In theory, quantum computers are able to solve some types of computations exponentially faster than classical computers. Shor’s algorithm to factor a number in its prime factors is often quoted as an example of this speedup. This opens the road to find cryptographic keys which form the backbone of today’s secure communication technologies. Once quantum computers become practical, this may well pose a threat to secure, encrypted communication. The key point here is that this threat requires a significantly larger quantum computer than is available now: for factoring a key of several thousand bits, a logical qubit register of several thousand qubits is required. With the current state-of-the-art, this requires millions of physical (noisy) qubits. Such a large quantum computer is at least 10 to 15 years away, if not more2.
Nevertheless, quantum computing is casting its shadow ahead. And governments and organisations are already taking countermeasures. Several governments now require all of their services to prepare for the security threat quantum computing will pose. It means that current encryption technologies are to be upgraded to a degree that even challenges quantum computing. This asks for longer encryption keys and more complex encrypting and decrypting algorithms, requiring more resources. Research and development of efficient digital cryptography systems, involving hardware and software, is already ongoing and will play an increasingly important role as quantum computers are coming of age3.
These observations lead to the conclusion that quantum computing will have an indirect impact on embedded software and beyond, in the next few years. It depends on the speed of evolution and innovation of quantum technology when quantum computing devices will leave the laboratory and make their introduction to the industry. For now, that appears to be a decade away, but vigilance on this subject is required. Europe should strive for independence from other nations in this area to be able to develop this technology on its own, in the light of the recent developments in international relations.
Quantum Internet
A quantum internet is an application of quantum networks. Quantum networks enable the communication of qubits. Such networks can be used to connect quantum processors to form more powerful quantum computers. Quantum networks can also be used to create quantum internet applications. One such application is the secure distribution of cryptographic keys: in this setup, cryptographic keys are distributed over a quantum network using entangled qubits, enabling the detection of eavesdropping on the communication. But quantum internet, just like quantum computers, are still in the research and development phase. Practical applications at this moment require complicated setups, often involving cryogenically-cooled devices, preventing wide-spread use today and in the next few years4.
Quantum Sensors
Quantum sensors are sensors which detect physical properties by using quantum effects such as quantum entanglement, quantum interference, and quantum state squeezing. Quantum sensors have been in use for quite a long time: medical magnetic resonance scanners, which detect the precession of atomic nuclei in a magnetic field.
Quantum sensors are sensitive to some physical property. It is not so much the measurement of the physical property, but the enhanced accuracy or sensitivity to such a property that makes quantum sensors stand out from classical sensors. As such, the (embedded) software that processes the measurement of quantum sensors does not differ from software that processes measurements from classical sensors5.
It appears that quantum technology will impact the communication security of embedded systems in the next few years. Implementations for post-quantum cryptography must be researched and developed to stay ahead of quantum technology developments.
Embedded software significantly improves the functionalities, features, and capabilities of ECPS, increasing their autonomy and efficiency, and exploiting their resources and computational power, as well as bringing to the field functionalities that used to be reserved only for data centres, or more powerful and resource-rich computing systems. Moreover, implementing specific functionalities in software allows for their re-use in different embedded applications due to software portability across different hardware platforms. Examples of increasing computational power of ECPS are video conferencing solutions: less than 20 years ago specialised hardware was still required to realise this function, with big screens in a dedicated set-up that could not be used for any other but a dedicated application. Today, video conferencing is available on every laptop and mobile phone, where the main functionality is implemented by software running on standard hardware. The evolution is pushing to the “edge” specific video conferencing functionalities, adopting dedicated and miniaturised hardware supported by embedded software (video, microphone, and speakers), thus allowing the ECS value chain to acquire a new business opportunity.
Following a similar approach, it has been possible to extend the functionalities of mobile phones and smart watches, which today can a.o. count steps, keep track of walked routes, monitor health, inform users about nearby restaurants, all based on a few extra hardware sensors and a myriad of embedded software applications. The trend is to replace specialised hardware applications with software running on generic computing hardware and supported by application-specific hardware, such as AI accelerators, neural chips. This trend is also contributing to the differentiation of the value creation downstream and upstream, as observed in the Advancy report 6 (see Figure 1.3.3).
These innovations require the following breakthroughs in the field of embedded software:
-
Increased engineering efficiency and an effective product innovation process (cf. Chapter 2.3 Architecture and Design: Methods and Tools).
-
Enabled adaptable systems by adaptable embedded software and machine reasoning.
-
Improved system integration and verification and validation.
-
Embedded software, and embedded data analytics and AI, to enable system health monitoring, diagnostics, preventive maintenance, and sustainability.
-
Data privacy and data integrity.
-
Model-based embedded software engineering and design as the basis for managing complexity in SoS (for the latter, cf. Chapter 2.3 Architecture and Design: Methods and Tools).
-
Improved multidisciplinary embedded software engineering and software: architecting/design for (systems) qualities, including reliability, trust, safety, security, overall system performance, installability, diagnosability, sustainability, and re-usability (for the latter, cf. Chapter 2.3 Architecture and Design: Methods and Tools and Chapter 2.4 Quality, reliability, safety and cybersecurity).
-
Upgradability, dealing with variability, extending lifecycle and sustainable operation.
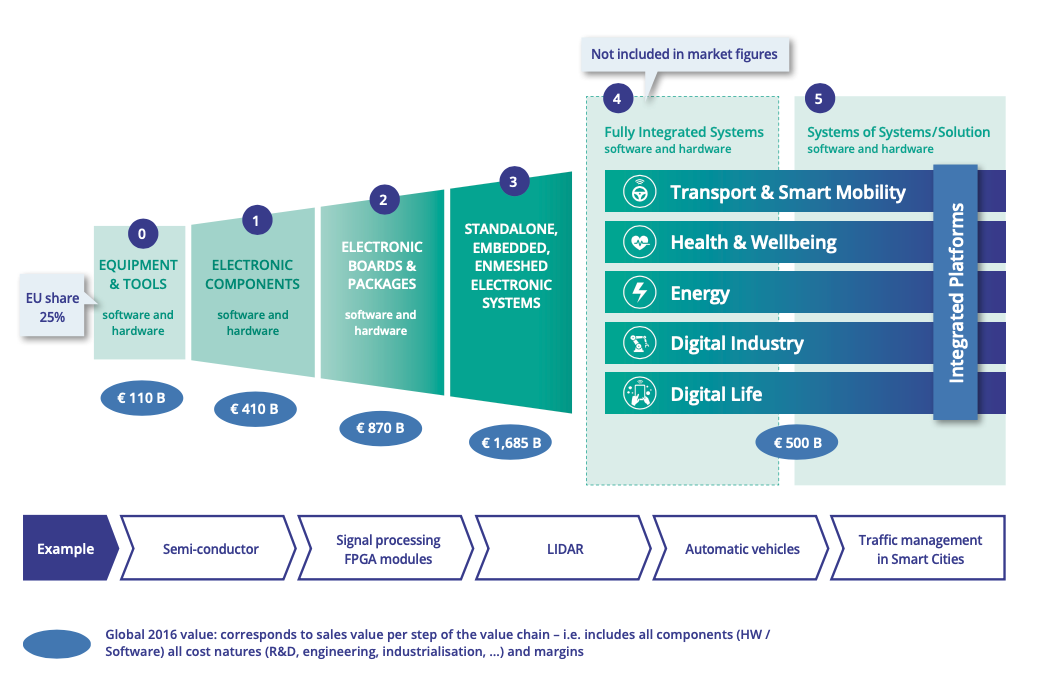
Research and innovation in the domain of embedded software and beyond will have to face seven challenges, each generated by the necessity for engineering automation across the entire lifecycle of sustainability, embedded intelligence and trust in embedded software.
-
Major Challenge 1: Efficient engineering of embedded software.
-
Major Challenge 2: Continuous integration and deployment.
-
Major Challenge 3: Lifecycle management.
-
Major Challenge 4: Embedding data analytics and artificial intelligence.
-
Major Challenge 5: Support for sustainability by embedded software.
-
Major Challenge 6: Software reliability and trust.
-
Major Challenge 7: Hardware virtualization for efficient SW engineering.
1.3.6.1 Major Challenge 1: Efficient engineering of embedded software
Embedded software engineering is frequently more a craft than an engineering discipline, which results in inefficient ways of developing embedded software. This is visible, for instance, in the time required for the integration, verification, validation and release of embedded software, which is estimated to exceed 50% of the total R&D&I expenses.
A new set of challenges to engineering embedded software is introduced with the emergence of heterogeneous computing architectures into the mainstream. It will be common for embedded systems to combine several types of accelerators to meet power consumption, performance requirements, safety, and real-time requirements. Development, optimisation, and deployment of software for these computing architectures proves to be challenging. If no solutions are introduced which automatically tailor software to specific accelerators8,9, developers will be overwhelmed with the required effort.
Software engineering is exceeding the human scale, meaning it can no longer be overseen by a human without supporting tools, in terms of velocity of evolution, and the volume of software to be designed, developed and maintained, as well as its variety and uncertainty of context. Engineers require methods and tools to work smarter, not harder, and need engineering process automation and tools and methods for continuous lifecycle support. To achieve these objectives, we need to address the following practical research challenges: shorter development feedback loops; improved tool-supported software development; methods and tools to enable strongly linked, yet independent and heterogenous development processes in new areas like software defined vehicles (SDV); empirical and automated software engineering; and safe, secure and dependable software platform ecosystems.
The demand of embedded software is higher than we can humanly address and deliver, exceeding human scale in terms of evolution speed, volume and variety, as well as in managing complexity. The field of embedded software engineering needs to mature and evolve to address these challenges and satisfy market requirements. In this regard, the following four key aspects must be considered.
-
From embedded software engineering to cyber physical systems engineering
Developing any high-tech system is, by its very nature, a multi-disciplinary project. There is a whole ecosystem of models (e.g. physical, mechanical, structural, (embedded) software and behavioural) describing various aspects of a system. While many innovations have been achieved in each of the disciplines separately, the entirety still works in silos, each with their own models and tools, and only interfacing at the borders between them. This traditional separation between the hardware and software worlds, and individual disciplines, is hampering the development of new products and services.
Instead of focusing only on the efficiency of embedded software engineering, we already see that the field is evolving into direction of cyber physical systems (cf. Chapter 2.3 Architecture and Design: Methods and Tools), and software is one element of engineering. Rather than silos and handovers at the discipline’s borders, we expect tools to support the integration of different engineering artefacts and enable, by default, effective development with quality requirements in mind – such as safety, security, reliability, dependability, sustainability, trustworthiness, and interoperability. New methods and tools will need to be developed to further facilitate software interaction with other elements in a system engineering context (cf. Chapter 2.3 Architecture and Design: Methods and Tools).
Software-defined systems enable the implementation of complex and customisable functionalities in CPS. The equivalent for the automotive industry is the software-defined vehicle (SDV), which often includes the concept of the connected vehicle and the associated cloud services. SDVs combine mechatronic - often safety-critical and real-time-capable - systems with edge, internet, app and cloud technologies. This requires the integration of embedded software (open and closed source), which in turn is often developed according to different paradigms, standards and business models. In the target vehicle system, these parts must not only function together, but also fulfill strict quality standards and, where applicable, legal regulations. SOA-based approaches are expected to contribute significantly towards these goals. Methods and tools are required that support individual but linked development cycles in the respective paradigms and at the same time enable the composition of the overall system, but also the decomposition of results from diagnostics, verification and validation back to the individual part.
Artificial intelligence is a technology that holds a great potential in dealing with large amounts of data, and potentially could be used for understanding complex systems. In this context, artificial intelligence has the potential to automate some daily engineering tasks, moving boundaries of type and size of tasks that are humanly possible in software engineering.
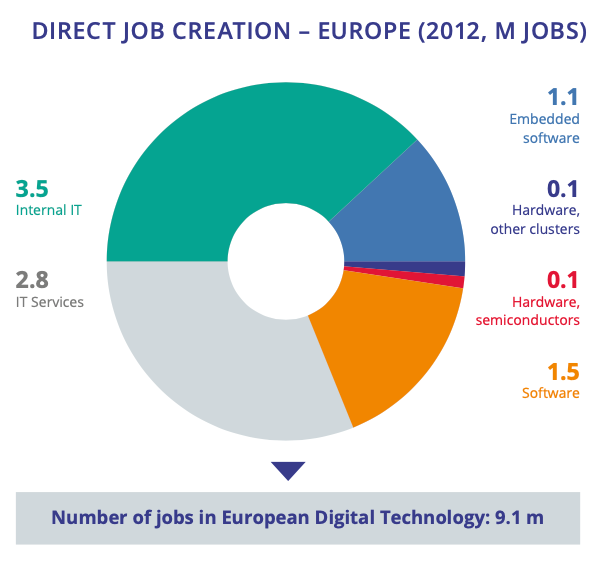
-
Software architectures for optimal edge computing
At the moment, Edge computing lacks proper definition and, including many different types of managed and unmanaged devices, this leads to uncertainty and difficulties on how to efficiently and effectively use software architectures, including aspects as resource, device, and network management (between edge devices as well between edge and fog/cloud), security, useful abstractions, privacy, security, reliability, and scalability. Additionally, automatic reconfiguration, adaptation and re-use face a number of challenges. These challenges are caused by diversity of edge devices and wide range of requirements in terms of Quality of Service (e.g. low latency, high throughput). In addition, sustainability and reliability are difficult to ensure when trying to prioritize between Quality of Service on the edge and end-to-end system Quality of Service.
Furthermore, the lack of definition also hampers the growing need for energy efficient computing and the development of energy consumption solutions and models across all layers from materials, via software architecture to embedded/application software. Energy efficiency is vital for optimal edge computing.
Lastly, as AI is also moving towards the edge (i.e., Edge AI) defining lightweight models and model architectures that can deal with low amounts of data available on the edge and still provide good model accuracy are desperately needed. Finally, this limits transfer of common solution patterns, best practices, and reference architectures, as Edge computing scope and configuration requires further clarification and classification.
Since edge devices need to be self-contained, edge software architectures need to support, from the one side, virtual machine-like architectures, and from the other side they need to support the entire software lifecycle. The fact that there are many different types of edge devices also requires an interoperability standard to ensure that they can work together. Innovations in this field should focus on, amongst others, software-hardware co-design, virtualisation and container technologies and new standard edge software architecture (middleware).
It is essential to discuss types of quality properties that become more significant as Edge computing is introduced, and based on these, build use cases that profit from quality properties specific to edge computing. There is a need for new approaches that enable early virtual prototyping of edge solutions, as well as approaches that enable verification and validation of quality properties during the entire life cycle of edge software systems. One of the possibilities for profiting from Edge is to focus on digital twins to monitor divergences from expected behaviour and implement logic that will benefit from Edge’s low latency when making critical decisions, especially in safety critical software systems.
-
Integration of embedded software
To ensure software development is more effective and efficient, it is necessary to place greater focus on integrating embedded software into a fully functional system. First, innovation in continuous system integration must include more effective ways of integrating legacy components into new systems (see also D). Second, for the integration of data and software, the embedded software running in the field has to generate data (such as on run-time performance monitoring, system health, quality of output, compliance to regulations, user interactions) that can be re-used to improve its quality and performance. By improving this, the data and software integration can not only improve the efficiency of embedded software itself, but also the internal coordination and orchestration between components of the system by ensuring a rapid feedback cycle. Third, it is paramount to enable closer integration of software with the available computing accelerators. This must be done in a way that frees developers from additional effort, while at the same time uses the full potential of heterogeneous computing hardware.
-
Using abstraction and virtualisation
The recent focus on model-driven (or “low-code”) software development has sparked a new approach to managing complexity and engineering software. Generating embedded software from higher-level models can improve maintainability and decrease programming errors, while also improving development speed. However, creating and managing models of real systems with an appropriate level of detail that allows for simulation and code generation is a challenge. Managing models and their variability is a necessity if we want to prevent shifting the code legacy problem to a model legacy problem where there are too many models with too much variety.
The core elements of the domain are captured in a language of the domain. The introduction of domain-specific languages (DSLs) and aspect-oriented languages has allowed for the inclusion of aspects and constructs of a target application domain into the languages used to develop embedded software. This abstraction allows for shortening the gap between software engineers and domain experts. We expect innovations in DSLs and tool support to establish a major boost in the efficiency of embedded software development.
The increased level of abstraction allows for more innovation in virtualisation of systems and is a step towards correctness by construction instead of correctness by validation/testing. Model-based engineering and digital twins of systems are already being used for a variety of goals – such as training, virtual prototyping and log-based fault analysis. Furthermore, they are necessary for supporting the transition towards sustainable ECPS. Innovations in virtualisation will allow DSLs to be (semi-)automatically used to generate digital twins with greater precision and more analysis capabilities, which can help us to explore different hardware and software options before a machine is even built, shortening development feedback loops due to such improved tool-supported software development.
-
Resolving legacy
Legacy software and systems still constitute most of the software running in the world today. It is only natural that the amount of legacy software increases in the future. While it is paramount to develop new and improved techniques for the development and maintenance of embedded software, we cannot ignore the systems currently in operation. New software developed with novel paradigms and new tools will not run in isolation, but rather have to be used increasingly in ecosystems of connected hardware and software, including legacy systems.
There are two main areas for innovation here. First, we need to develop efficient ways of improving interoperability between new and old. With years of development, and a need to continue operations, we will have to depend on legacy software for the foreseeable future. It is therefore imperative to develop new approaches to facilitating reliable and safe interactions, including wrapping old code in re-usable containers. Second, we must innovate the process to (incrementally) migrate, rejuvenate, redevelop and redeploy legacy software, both in isolation and as part of a larger system. We expect innovations in these areas to increase efficiency and effectiveness in working with legacy software in embedded software engineering.
The key focus areas in the domain of efficient embedded software engineering include the following. There is a strong relation between Major Challenges 1, 2, 3, and 6 below, and Chapter 2.3 "Architectures and Design: Methods and Tools“, specifically Major Challenges 1 and 2.
-
Model-based software engineering:
-
Model-based software engineering enabling systems to become part of SoS.
-
Model inference to enable re-use, refactoring and evolution of existing subsystems in SoS.
-
Model-based testing that takes the re-use of uncontrolled systems into account.
-
Embedded software architectures to facilitate building SoS.
-
-
Digital twinning:
-
Virtualisation as a means for dealing with legacy systems.
-
Virtualisation and virtual integration testing (using Digital Twins and specialized design methods, like, e.g. contract-based design, for guaranteeing safe and secure updates (cf. Architecture and Design: Methods and Tools Chapter 2.3).
-
Approaches to reduce re-release/re-certification time, e.g. model-based design, contract-based design, and modular architectures.
-
Distinct core system versus applications and services.
-
Design for X (e.g design for test, evolvability and updateability, diagnostability, and adaptability).
-
-
Constraint environments:
-
Knowledge-based leadership in design and engineering.
-
Resource planning and scheduling (including multi-criticality, heterogeneous platforms, multicore, software portability).
-
Simulation and Design for software evolution over time, while catering for distinct phases.
-
Exploiting hybrid compute platforms, including efficient software portability.
-
-
Software technology:
-
Virtualisation as a tool for efficient engineering.
-
Interface management enabling systems to become part of SoS.
-
Technology for safe and dependable software ecosystems.
-
Artificial intelligence-based tools to support software engineering, software production and testing efforts.
-
Co-simulation platforms.
-
-
SW engineering tools:
-
Integrating embedded AI in software architecture and design.
-
Programming languages for developing large-scale applications for embedded systems.
-
Models & digital twins, also at run-time for maintainability and sustainability.
-
Programming models, compilers, code generators, and frameworks for optimal use of heterogeneous computing platforms.
-
Co-simulation platforms.
-
Tools, middleware and (open) hardware with permissible open-source licenses.
-
Methods and tools that support individual but linked development cycles in the respective paradigms (safety, real-time, edge/cloud, open/closed source) and velocities on the one hand, and integration, verification and validation in complex target systems like SDV on the other hand.
-
1.3.6.2 Major Challenge 2: Continuous integration and deployment
It is fair to assume that most future software applications will be developed to function as part of a certain platform, and not as stand-alone components. In some embedded system domains, this idea has been a reality for a decade (e.g. in the AUTomotive Open System Architecture (AUTOSAR) partnership, which was formed in 2003). Increasingly the platforms have to support SoS and IoT integration and orchestration, involving a large amount of diverse small devices. Guaranteeing quality properties of software (e.g. safety and security) is a challenging task, and one that only becomes more complex as the size and distribution of software applications grow, especially if software is not properly designed for its intended operational context (cf. Chapter 2.3 Architecture and Design: Methods and Tools). Although we are aiming towards continuous integration on the level of IoT and SoS, we are still struggling with the integration of code changes from multiple contributors into a single software system.
One aspect of the problem relates to the design of SoS10, which are assumed to be composed of independent subsystems but over time have become dependent. Orchestration between the different subsystems, that may involve IoT as well, is an additional issue here. Another aspect relates to the certification of such systems that requires a set of standards. This applies especially for IoT and SoS and it is complicated by the introduction of AI into software systems. Although AI is a software-enabled technology, there are still many issues on the system level when it comes to its integration into software systems. It is particularly challenging to ensure their functional safety and security, and thus to certify such systems. Some of the existing initiatives include, e.g. for vehicles, ISO 21448 (Road Vehicles – Safety Of The Intended Functionality (SOTIF)), ISO/TR 4804 (followed by ISO/AWI TS 5083, currently in development), ANSI/UL 4600 Standard for Safety for the Evaluation of Autonomous Products, and SAE J3016, which recommends a taxonomy and definitions for terms related to automated driving. Note, that AI may be applied as an engineering tool to simplify certification.
Finally, integration and delivery practices are part of the engineering processes. Although methodologies already exist to achieve this (such as DevSecOps and ChatOps), these mostly relate to software production. With ECPS, continuous integration becomes increasingly more complex due to the wide range of hardware architectures and platforms, each with its own unique characteristics. Continuous integration must account for this diversity, requiring cross-compilation and testing on various target devices. Continuous delivery must deal with the fact that the products into which the new software modules have to be delivered are already sold and ‘working in the field’, often in many different variants (i.e. the whole car fleet of an OEM). Even in domains where the number of variant systems is small, retaining a copy of each system sold at the producing company in order to have a reference target is prohibitive. Thus, virtual integration using model-based design methods (including closed-box models for legacy components) and digital twins used as integration targets as well as for verification & validation by physically accurate simulation are a mandatory asset for any system company to manage the complexity of ECPS and their quality properties. System engineering employing model-based design and digital twins must become a regular new engineering activity.
Europe is facing a great challenge with the lack of platforms that are able to adopt embedded applications developed by individual providers into an ecosystem (cf. Reference Architectures and Platforms in Chapter 2.3). The main challenges here are to ensure the adequate functionality of integrated systems (which is partially solved by the micro-services approach), while ensuring key quality properties such as performance, safety, and security (see also Major Challenge 6), which is becoming increasingly complex and neglected as we adopt approaches that facilitate only integration on the functional level. Instrumental for these challenges is the use of integration and orchestration platforms that standardise many of the concerns of the different parts in the SoS, some of which are connected via IoT. In addition, Automated engineering processes such as CI/CD will be crucial to adapt. The primary DevOps methodology needs to be adjusted for the ECPS. For example, CI pipelines will enable toolchain selection configuration for cross-compiling, or they will include real-time testing and validation, which can be more challenging to automate and verify. Integrating automated tests for hardware interactions can be complex and require specialized hardware-in-the-loop (HIL) testing setups.
ECPS will become a part of an SoS and eventually SoECPS. SoS challenges like interoperability, composability, evolvability, control, management and engineering demand ECPS to be prepared for a life as a part of a SoS (cf. Chapter 1.4 System of systems). Thus, precautions at individual ECPS's are necessary to enable cost efficient and trustworthy integration into SoS.
Therefore, it is essential to tackle these challenges by good engineering practices: (i) providing sets of recommended code and (system to system) interaction patterns; (ii) avoiding anti-patterns; and (iii) ensuring there is a methodology to support the integration from which the engineers of such systems can benefit. This implies aiming to resolve and pre-empt as many as possible of the integration and orchestration challenges at the platform design level. It also involves distribution of concerns to the sub systems in the SoS or IoT. Followed by automated engineering processes applying the patterns and dealing with the concerns in standardised ways. Besides this, it is necessary to facilitate communication between different stakeholders to emphasise the need for quality properties of ECPS, and to enable (automated) mechanisms that raise concerns sufficiently early to be prevented, while minimising potential losses.
On the development level, it is key to enhance the existing software systems development methodologies to support automatic engineering, also to automate the validation and verification processes for new features as they are being introduced into the system. This might need the use of AI in the validation and verification process. At this level, it is also necessary to use software system architectures in the automation of verification and other engineering practices, to manage the complexity that arises from such integration efforts (also see Major Challenge 3 below).
Artificial Intelligence and Machine Learning
Progress in AI keeps being fast-paced. While typical ECPS-needs such as Computer Vision are dominated by AI since quite some time, recent progress in Large Language Models (LLMs) opened the door to serious AI-assisted engineering tools. Hence, AI is on the way to becoming an important tool for the engineering of Embedded Software, while being an essential part of Embedded Software at the same time.
AI in Embedded Software. Simply speaking, AI lets us implement functions we don’t need to understand as they are learned automatically. This has advantages and drawbacks. We take advantage of this property in tiny sensors, where AI (e.g. TinyML) automatically approximates a mapping of property changes of some material to a measurement value, in prediction, control or virtual sensing, where AI has learned to interpret time series, images, or other forms of data, and many other tasks, including detection and tracking of objects. AI functions can also serve as (automated) abstractions. With this versatility, wide-spread adoption, and a potential non-understanding of what was learned, come challenges like fitting the AI functionality to the available resources (cf. RISC-V with research on AI accelerators), testability / explainability / trustworthiness / integration in safety critical applications, real-time performance, updateability / continuous learning / maintainability, and more. In general, AI in Embedded Software faces all the same challenges standard Embedded Software has, some in a more demanding form of course. For example, using sub-symbolic AI in a safety-critical context is without a clear-cut solution regarding the trustworthiness issues. To compensate for these, extensive monitoring, and the implementation of certain architectures (e.g. Simplex) might be necessary. This shows that relying on AI in a system will have a big impact on the system design. Relying on AI will also have a massive impact on verification planning and software engineering as, e.g. proper training and validation data sets need to be provided.
AI for Embedded Software engineering. Software engineering is the discipline of building software in a proper way. AI can help with that, and AI functionality has been explored in tools for software engineering for some time. However, up to now AI-algorithms were mostly used in tools for verification (automated test case generation, checking) and less so in others. Recent developments in LLMs demonstrate the huge potential for using AI in areas like model or code generation from natural language, refactoring or “rejuvenation” of legacy software, porting software while preserving investment, or automatically adapting software to different settings/platforms. Like before, the major challenge is that there is no guarantee on the correctness or fit-for-purpose on the output of these LLMs, which is a major research opportunity at the same time. In future, AI is likely to replace many of the software-coding activities done by engineers today. Also, domain specific models will speed-up software design & generation by a considerable amount, and AI will support engineers in system understanding and in mastering complexity.
Summing up, AI hasn’t yet shown its full potential for the use in and around Embedded Software – there are still many challenges left as demanding research topics. However, based on the current state-of-art and results, it is clear that AI will be a core part of future Embedded Software and ECPS.
The RISC-V instruction set architecture (ISA) is the fifth version of the Berkeley ISA that has seen exponential commercial and academic adoption in the last 10 years thanks to its open-source nature, as well as its modularity, extendibility, and simple architecture. Many commercial users adopt RISC-V in their System-On-Chips, from both open-source repositories (where the Register-Transfer-Level description of the RISC-V CPU is published), or from closed-source IP vendors. The increase in silicon devices together with the need for digital sovereignty caused many national and international organizations to take action, and RISC-V is a main actor of this revolution. For example, the European Commission11 is building an open-source ecosystem to expand its innovation on RISC-V to compete with existing commercial alternatives, covering hardware design and system-on-chips, all the way to electronic design automation tools and the full software stack. In this scenario, OpenHW Group12 and the Eclipse Foundation13 play a key role in developing open-source, high-quality, silicon-proven RISC-V IPs under a permissive license. Both the not-for-profit foundations are driven by their members, who invested in RISC-V and open-source-based System-On-Chip. The modularity and extendability of the RISC-V ISA allow users to design their own architecture to meet the ultra-low-power and energy-efficient edge-computing devices constraints, as well as high-performance server machines requirements. For example, the “RVV RISC-V Vector” ISA extension allows to process multiple data concurrently, or custom extensions for security to encrypt data more efficiently. For this reason, it is crucial to have a holistic software stack to cope with the wide range of applications, taking into account the deployment of efficient applications leveraging the different ISA extensions and computer architectures.
The key focus areas identified for this challenge include the following:
-
Continuous integration of embedded software:
-
Model based design and digital twins to support system integration (HW/SW) and HW/SW co-development (increasingly new technologies have to be integrated).
-
Applying automation of engineering, taking architecture, platforms and models into account.
-
Virtualisation and simulation as tools for managing efficient integration and validation of configurations, especially for shared resources and other dependability issues.
-
Application of integration and orchestration practices to ensure standard solutions to common integration problems.
-
Integration and orchestration platforms and separation of concerns in SoS and IoT.
-
Enabling reliable and safe continuous SW delivery to already working devices.
-
-
Verification and validation of embedded software:
-
(Model) test automation to ensure efficient and continuous integration of CPSs.
-
Enabling secure and safe updates (cf. Major Challenge 3) and extending useful life (DevOps).
-
Continuous integration, verification and validation (with and without AI) enabling continuous certification with automated verification & validation (especially the focus on dependability), using model-based design technologies and digital twins; also when SoS and IoT are involved.
-
Certification of safety-critical software in CPSs.
-
1.3.6.3 Major Challenge 3: Lifecycle management
Complex systems such as airplanes, vehicles and medical equipment are expected to have a long lifetime, often up to 30 years. The cost of keeping these embedded systems up to date, making them relevant for the everyday challenges of their environment is often time-consuming and costly. This is becoming more complex due to the fact that most of these systems are cyber-physical systems, meaning that they link the physical world with the digital world, and are often interconnected with each other or to the internet. With more and more functionalities being realized by embedded software, over-the-air updates – i.e. deploying new, improved versions of software-modules unto systems in the field – become an increasingly relevant topic. Apart from updates needed for error and fault corrections, performance increases and even the implementation of additional functionalities – both optional or variant functionalities that can be sold as part of end-user adaptation as well as completely new functionalities that are needed to respond to newly emerging environmental constraints (e.g. new regulations, new features of cooperating systems). Such update capabilities perfectly fit and are even required for the ‘continuous development and integration’ paradigm.
Embedded software also must be maintained and adapted over time, to fit new product variants or even new product generations and enable updateability of existing systems. If this is not effectively achieved, the software becomes overly complex, with prohibitively expensive maintenance and evolution, until systems powered by such software are no longer sustainable.
We must break this vicious cycle and find new ways to create software that is long-lasting and which can be cost-efficiently evolved and migrated to use new technologies. Practical challenges that require significant research in software sustainability include: (i) organisations losing control over software; (ii) difficulty in coping with modern software’s continuous and unpredictable changes; (iii) dependency of software sustainability on factors that are not purely technical; (iv) enabling “write code once and run it anywhere” paradigm.
As software complexity increases, it becomes more difficult for organisations to understand which parts of their software are worth maintaining and which need to be redeveloped from scratch. Therefore, we need methods to reduce the complexity of the software that is worth maintaining and extracting domain knowledge from existing systems as part of the redevelopment effort. This also relates to our inability to monitor and predict when software quality is degrading, and to accurately estimate the costs of repairing it. Consequently, sustainability of the software is often an afterthought. This needs to be flipped around – i.e. we need to design “future-proof” software that can be changed efficiently and effectively, or at least platforms for running software need to either enable this or force such way of thinking.
As (embedded) software systems evolve towards distributed computing, SoS and microservice-based architectural paradigms, it becomes even more important to tackle the challenges of integration at the higher abstraction levels and in a systematic way. Especially when SoS or IoT is involved, it is important to be able to separate the concerns over the subsystems.
The ability of updating systems in the field in a way that safety of the updated systems as well as security of the deployment process is maintained will be instrumental for market success of future ECPS. Over-The-Air solutions become key enablers to this regard, especially for distributed systems, and they will have to cover the different paces at which HW and SW evolve, determining when updates become necessary. Edge-to-cloud continuum represents an opportunity to create software engineering approaches and engineering platforms that together enable deployment and execution of the same code anywhere on this computing continuum.
The ability of keeping track of system parameters like interface contracts and composability requires a framework to manage these parameters over the lifetime. This will enable the owner of the system to identify at any time how the system is composed and with what functionality. To this regard, the onboarding process of the constituent systems becomes a crucial phase to maintain the desired levels of security and safety: SoS integration platforms should provide solid onboarding procedures that guarantee no compromised HW and SW become part of the SoS. The adoption of block chain technologies, digital contracts and security certificates, etc. could prevent similar situations, which could impact not only the SoS during operation but also the entire supply chain associated to it. The onboarding phase should also be automated to increase security levels and ensure scalability.
Instead of focusing just on the efficiency of embedded software engineering, we already see that the field is evolving into the direction of cyber physical systems (cf. Chapter 2.3 Architecture and Design: Methods and Tools), and software is one element of engineering.
Many software maintenance problems are not actually technical but people problems. There are several socio-technical aspects that can help, or hinder, software change. We need to be able to organise the development teams (e.g. groups, open-source communities) in such a way that it embraces change and facilitates maintenance and evolution, not only immediately after the deployment of the software but for any moment in the software lifecycle, for the decades that follow, to ensure continuity. We need platforms that are able to run code created for different deployment infrastructures, without manual configuration.
The expected outcome is that we are able to keep embedded systems relevant and sustainable across their complete lifecycle, and to maintain, update and upgrade embedded systems in a safe and secure, yet cost-effective way.
The key focus areas identified for this challenge include the following.
-
Rejuvenation of systems:
-
Software legacy and software rejuvenation to remove technical debt (e.g. software understanding and conformance checking, automatic redesign and transformation).
-
Continuous platform-agnostic integration, deployment and migration.
-
End-of-life and evolving off-the-shelve/open source (hardware/software).
-
-
Managing complexity over time:
-
Interplay between legacy software and new development approaches.
-
Vulnerability of connected systems.
-
Continuous certification of updates in the field (reduce throughput time).
-
Intelligent Diagnostics of systems in the field (e.g. guided root cause analysis).
-
-
Managing configurations over time:
-
Enable tracking system configurations over time.
-
Create a framework to manage properties like composability and system orchestration.
-
-
Evolvability of embedded software:
-
Technology, including automation of engineering and the application of integration and orchestration platforms, for keeping systems maintainable, adaptable and sustainable considering embedded constraints with respect to resources, timing and cost: new functionalities enabling and facilitating secure and automated onboarding processes, OTA software maintenance (see also the SoS chapter), ….
-
Embedded software architectures to enable SoS.
-
1.3.6.4 Major Challenge 4: Embedded Artificial Intelligence
For various reasons – including privacy, energy efficiency, latency and the increasing necessity of smart data analytics on site – processing and artificial intelligence are moving towards the edge (edge computing), forcing the software stacks of embedded systems to coherently evolve supporting these new computing paradigms. As detailed in the Chapter “Edge Computing and Embedded Artificial Intelligence”, non-functional constraints of embedded systems, such as timing, energy consumption, low memory and computing footprint, being tamperproof, etc., need to be taken into account compared to software with similar functionalities when migrating these from the cloud to the edge. Furthermore, the Quality, Reliability, Safety and Security Chapter states that key quality properties when embedding of AI components in digitalized ubiquitous systems are determinism, understanding of nominal and degraded behaviours of the system, their certification and qualification, and clear liability and responsibility chains in the case of accidents. When engineering software contains AI-based solutions, it is important to understand the challenges that such solutions introduce. Indeed, AI contributes to address challenges of embedded software, but it does not define them exclusively itself, as quality properties of embedded software depend on integration of AI-based components with other software components.
For efficiency reasons, very intensive computing tasks (such as those based on deep neural networks, DNNs) are being carried out by various accelerators embedded in systems on a chip (SoCs). Although the “learning” phase of a DNN is still mainly done on big servers using graphics processing units (GPUs), local adaptation is moving to edge devices. Also, LLMs are experiencing a similar evolution towards the edge. Alternative approaches, such as federated learning, allow for several edge devices to collaborate in a more global learning task. Therefore, the need for computing and storage is ever-increasing, and is reliant on efficient software support.
The “inference” phase (i.e. the use after learning) is also requiring more and more resources because neural networks are growing in complexity exponentially. Once carried out in embedded GPUs, this phase is now increasingly performed on dedicated accelerators. Most middle and high-end smartphones have SoCs embedding one of several AI accelerators, as well as Mx Apple processors family for laptops, tablets and future wearables – for example, the Nvidia Jetson Xavier NX is composed of six Arm central processing units (CPUs), two inference accelerators, 48 tensor cores and 384 Cuda cores. Obtaining the best of the heterogeneous hardware is a challenge for the software, and the developers should not have to be concerned about where the various parts of their application are running.
Once developed (on servers), a neural network has to be tuned for its embedded target by pruning the network topology using less precision for operations (from floating point down to 1-bit coding) while preserving accuracy. This was not a concern for the “big” AI development environment providers (e.g. Tensorflow, PyTorch, Caffe2, Cognitive Toolkit) until recently. This has led to the development of environments14 designed to optimise neural networks for embedded architectures15 to move towards the Edge.
Most of the time the learning is done on the cloud. For some applications/domains, making a live update of the DNN or LLM characteristics is a sought-after feature, including all the risks of security, interception. Imagine the consequences of tampering with the DNN or LLM used for a self-driving car! A side-effect of DNN or LLM is that intellectual property is not in a code or algorithm, but rather lies in the network topology and its weights, and therefore needs to be protected.
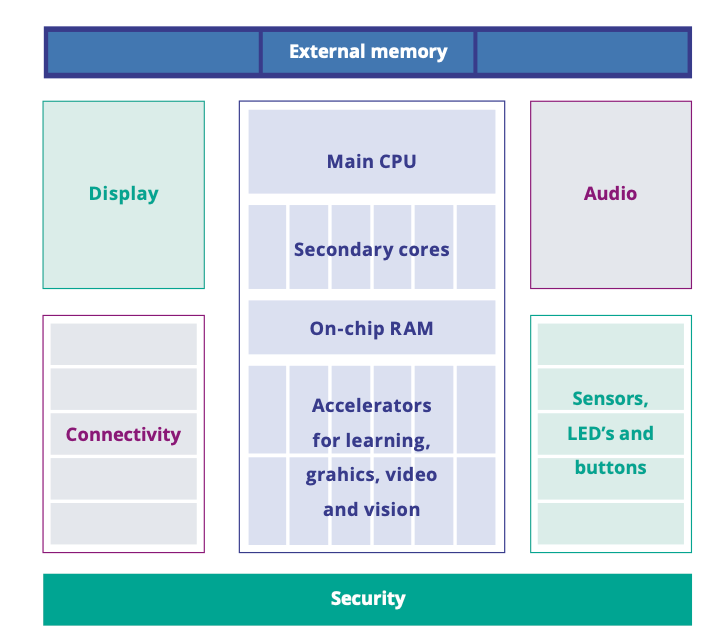
European semiconductor companies lead a consolidated market of microcontrollers and low-end microprocessors for embedded systems, but are increasing the performance of their hardware, mainly driven by the automotive market and the increasing demand for more performing AI for advanced driver-assistance systems (ADAS) and self-driving vehicles. With edge computing and embedded AI this trend has extended to other vertical domains that are key for Europe. European semiconductor companies are also moving towards greater heterogeneity by adding specialised accelerators. On top of this, Quality, Reliability, Safety and Security Chapter lists personalization of mass products and resilience to cyber-attacks, as the key advantage and the challenge characterizing future products. Embedded software needs to consider these and find methods and tools to manage their effects on quality properties of software that integrates them. Also, embedded software engineering will need to ensure interoperability between AI-based solutions and non-AI parts.
In this context, there is a need to provide a programming environment and libraries for the software developers. A good example here is the interchange format ONNX, an encryption format for protection against tampering or reverse engineering that could become the foundation of a European standard. Beside this, we also need efficient libraries for signal/image processing for feeding data and learning into the neural network, abstracting from the different hardware architectures. These solutions are required to be integrated and embedded in ECPS, along with significant effort into research and innovation in embedded software.
The key focus areas identified for this challenge include the following.
-
Federated and distributed learning:
-
Create federated learning at the edge in heterogeneous distributed systems (analysis, modelling and information gathering based on local available information).
-
Federated intelligence at the edge (provide context information and dependability based on federated knowledge).
-
-
Embedded Intelligence:
-
Create a software AI framework to enable reflecting and acting on the systems own state.
-
Dynamic adaption of systems when environment parameters and sensors like IoT devices are changing.
-
-
Data streaming in constraint environments:
-
Feed streaming data into low-latency analysis and knowledge generation (using context data to generate relevant context information).
-
-
Embedding AI accelerators:
-
Accelerators and hardware/software co-design to speed up analysis and learning (e.g. patter analysis, detection of moves (2D and 3D) and trends, lighting conditions, shadows).
-
Actual usage-based learning applied to accelerators and hardware/software co-design (automatic adaptation of parameters, adaptation of dispatch strategies, or use for new accelerators for future system upgrades).
-
1.3.6.5 Major Challenge 5: Support for sustainability by embedded software
The complete power demand in the whole ICT market currently accounts from 5% to 9% of the global power consumption16. The ICT electricity demand is rapidly increasing and it could go up to nearly 20% in 2030. Compared to estimated power consumption of future large data centres, embedded devices may seem to be a minor problem. However, when the devices are powered by batteries, they still have a significant environmental impact. Energy efficient embedded devices produce less hazardous waste and last longer without the need for replacement.
The growing demand for ultra-low power electronic systems has motivated research into device technology and hardware design techniques. Experimental studies have proven that the hardware innovations for power reduction can be fully exploited only with proper design of the upper layer software. Partitioning hardware enables smart power-up/power-down strategies. Together with support through resource-aware algorithms, this could lead to significant energy savings. The same applies to software power and energy modelling and analysis: the first step towards the energy reduction is complex due to the inter- and intra-dependencies of processors, operating systems, application software, programming languages and compilers. Software design and implementation should be viewed from a system energy conservation angle rather than as an isolated process.
For sustainability, it is critical to understand quality properties of software. These include in the first place power consumption, and then other related properties (performance, safety, security, and engineering-related effort) that we can observe in the context of outdated or inadequate software solutions and indicators of defected hardware. Power reduction strategies are mainly focusing on processing, storage, communication, and sometimes on other (less intelligent) equipment.
For the future embedded software developers, it is crucial to keep in touch with software development methodologies focused on sustainability, such as green computing movement, resource-aware computing and, more generally, sustainable programming techniques. In the domain of embedded software, examples include the estimation of the remaining useful life of the device, the network traffic and latency time optimization, the process scheduling optimization or energy efficient workload distribution, the management of HW and SW resources oriented to energy saving, the correct use of software abstraction, etc.
The concept of sustainability is based on three main pillars: ecological, economic and social. The ideal environmentally sustainable (or green) software in general requires as little hardware as possible, it is efficient in power consumption, and its usage leads to minimal waste production. Embedded software designed to be adaptable for future requirements without the need to be replaced by a completely new product is an example of environmentally, economically, and socially sustainable software.
To reach the sustainability goal, the embedded software design shall focus also on energy-efficient design methodologies and tools, energy efficient and sustainable techniques for embedded software and systems production and to the development of energy- and resource-aware applications and frameworks for embedded systems, edge computing, embedded intelligence and their applications.
It is evident that energy/power management has to be analysed with reference to the context, to the underlying hardware resources and the overall system functionalities. The coordinated and concentrated efforts of a system architect, hardware architect and software architect should help to introduce energy-efficient systems (cf. Chapter 2.3, Architecture and Design: Methods and Tools). The tight interplay between energy-oriented hardware, energy-aware and resource-aware software calls for innovative structural, functional and mathematical models for analysis, design and run-time. Model-based software engineering practices, supported by appropriate tools, will definitely accelerate the development of modern complex systems operating under severe energy constraints. It is crucial to notice the relationship between power management and other quality properties of software systems (e.g. under certain circumstances it is adequate to reduce the functionality of software systems by disabling certain features, which results in significant power savings). From a complementary perspective, when software is aware of the available hardware resources and their energy profile, it enables power consumption optimisation and energy saving, being able to configure the hardware resources, to activate/deactivate specific hardware components, increase/decrease the CPU frequency according to the processing requirements, partition, schedule and distribute tasks.
Therefore, in order to enable and support sustainability through software, software solutions need to be reconfigurable in the means of their quality. There must exist strategies for HW/SW co-design and accelerators to enable such configurations, and the entire integrated development environment should facilitate a rational use of abstraction (abstraction simplifies software development but increases the energy consumption) and supports HW and SW resources usage optimisation from the energy perspective directly from the programming language and through the compiler, linker, assembler, etc. For this to be possible, software systems need to be accompanied with models of their quality properties and their behaviour, including the relationship between power consumption and other high level quality properties. This will also enable balancing mechanisms between local and remote computations to reduce communication and processing energy consumption.
Models (digital twins) should be aware of energy use, energy sources, HW and SW resources and their sustainability profile. An example of this in SoS are solar cells that give different amounts of energy dependent on time of day and weather conditions.
The following key focus areas have been identified for this challenge:
-
Resource-aware software engineering.
-
Tools and techniques enabling the energy-efficient and sustainable embedded software design.
-
Development of energy-aware and sustainable frameworks and libraries for embedded software key application areas (e.g. IoT, Smart Industry, wearables, etc.).
-
Management of computation power on embedded hardware:
-
Management of energy awareness of embedded hardware, embedded software with respect to, amongst others, embedded high-performance computing (HPC).
-
-
Composable efficient abstractions that drive sustainable solutions while optimising performance:
-
Enabling resource-aware computing.
-
Enabling technologies for the second life of (legacy) cyber-physical systems.
-
Establish relationships between power consumption and other quality properties of software systems, including engineering effort (especially in cases of computing-demanding simulations).
-
Digital twins can support the management of quality properties of software with the goal of reducing power consumption, as the major contributing factor to the green deal, enabling sustainability.
-
1.3.6.6 Major Challenge 6: Software reliability and trust
Two emerging challenges for reliability and trust in ECPS relate to computing architectures and the dynamic environment in which ECPS exist. The first challenge is closely related to the end of Dennard scaling17. In the current computing era, concurrent execution of software tasks is the main driving force behind the performance of processors, leading to the rise of multicore and manycore computing architectures. As the number of transistors on a chip continues to increase (Moore’s law is still alive), industry has turned to a heavier coupling of software with adequate computing hardware, leading to heterogeneous architectures. The reasons for this coupling are the effects of dark silicon18 and better performance-to-power ratio of heterogeneous hardware with computing units specialised for specific tasks. The main challenges for using concurrent computing systems in embedded systems remain: (i) hard-to-predict, worst-case execution time; and (ii) testing of concurrent software against concurrency bugs19.
The second challenge relates to the dynamic environment in which ECPS execute. On the level of systems and SoS, architectural trends point towards platform-based designs – i.e. applications that are built on top of existing (integration and/or middleware) platforms. Providing a standardised “programming interface” but supporting a number of constituent subsystems that is not necessarily known at design time, and embedding reliability and trust into such designs, is a challenge that can be solved only for very specialised cases. The fact that such platforms – at least on a SoS level – are often distributed further increases this challenge.
On the level of systems composed from embedded devices, the most important topics are the safety, security, and privacy of sensitive data. Security challenges involve: (i) security of communication protocols between embedded nodes, and the security aspects on the lower abstraction layers; (ii) security vulnerabilities introduced by a compiler20 or reliance on third-party software modules; and (iii) hardware-related security issues21. It is necessary to observe security, privacy and reliability as quality properties of systems, and to resolve these issues on a higher abstraction level by design22, supported by appropriate engineering processes including verification (see Chapters 2.3 and 2.4).
European industry today relies on developed frameworks that facilitate production of highly complex embedded systems (for example, AUTOSAR in the automotive industry).
The ambition here is to reach a point where such software system platforms are mature and available to a wider audience. These platforms need to enable faster harvesting of hardware computing architectures that already exist and provide abstractions enabling innovators and start-ups to build new products quickly on top of them. For established businesses, these platforms need to enable shorter development cycles while ensuring their reliability and providing means for verification & validation of complex systems. The purpose of building on top of these platforms is ensuring, by default, a certain degree of trust for resulting products. This especially relates to new concurrent computing platforms, which hold promise of great performance with optimised power consumption. Recent developments in programming languages - such as Rust - look promising, as they aim to solve some of these inherited problems by default, based on available programming language constructs.
Besides frameworks and platforms that enable easy and quick development of future products, the key enabler of embedded software systems is their interoperability and openness. In this regard, the goal is to develop and make software libraries, software frameworks and reference architectures which need to ensure, by design, the potential for monitoring, verifying, testing and auto-recovering of embedded systems. That enables interoperability and integration of products developed on distributed computing architectures available to a wider audience. One of the emerging trends to help achieving this is the use of digital twins. Digital twins are particularly suitable for the verification of safety-critical software systems that operate in dynamic environments. However, development of digital twins remains an expensive and complex process, which has to be improved and integrated as part of the standard engineering processes (see Major Challenge 2 in Chapter 2.3).
We envision an open marketplace for software frameworks, middleware, and digital twins that represents a backbone for the future development of products. While such artefacts need to exploit the existing software stacks and hardware, they also need to support correct and high-quality software by design. Special attention is required for Digital Twin simulations of IoT devices to ensure reliability and trust in operating in real life.
Focus areas of this challenge are related to quality aspects of software. For targets such as new computing architectures and platforms, it is crucial to provide methodologies for development and testing, as well as for the team development of such software. These methodologies need to take into account the properties, potentials and limitations of such target systems, and support developers in designing, analysing and testing their implementations. As it is fair to expect that not all parts of software will be available for testing at the same time, it is necessary to replace some of the concurrently executing models using simulation technologies. Finally, these achievements need to be provided as commonly available software modules that facilitate the development and testing of concurrent software.
New concepts for programming languages, such as Rust, by their default design resolve some of the listed issues in developing computing architectures. For example, one of the main goals of Rust is handling concurrent and parallel programming in a safe and efficient way23. New concepts in the area of programming languages need to balance between several factors. From one side, new programming languages need to offer higher productivity to engineers. Programming languages need to enable more efficient collaboration between engineers by being more suitable to higher level architectural thinking that prioritise decoupled development of individual software components. Furthermore, traditional programming languages, such as C and C++, are challenging for static analysis due to undecidability of their statements24 and in general have huge test space making them susceptible to security issues. Finally, new languages need to solve by design complex problems in programming, such as concurrency and parallelism. On the other hand, it is necessary to minimize overhead of abstractions in new programming languages (performance25 and energy26 27 overhead). Besides creating such languages or extending the existing ones with news features, it is still necessary to provide methodologies that will guide industry to migrate their code bases to new programming constructs, or at least ensure co-existence and interaction between new and old code bases. With the introduction of AI into software engineering, we are looking for programming languages that will facilitate a new AI-assisted28 software development approach. That means programming languages that engineers can use to easily and as deterministically as possible express their intentions, while those languages are suitable for AI to enable code generation and automatization of validation, verification, and testing activities. Furthermore, we hope to have in the future such programming languages that facilitate, with the assistance from AI, analysis of quality properties of whole software stacks (e.g., WCET analysis, safety analysis29).
The next focus area is testing of systems against unexpected uses, which mainly occurs in systems with a dynamic execution environment. It is important here to focus on testing of self-adapting systems where one of the predominant tools is the simulation approach, and more recently the use of digital twins.
However, all these techniques are not very helpful if the systems are not secure and reliable by design. Therefore, it is necessary to investigate platforms towards reliability, security and privacy, with the following challenges.
-
Reliable software on new hardware including edge, fog and cloud processing: (co) verification of distributed, also heterogenous systems.
-
Verification and validation of ML models.
-
Robustness against unexpected uses:
-
Trustworthy, secure, safe, privacy-aware.
-
Validating self-adapting systems for example through simulation.
-
-
Security and privacy as a service:
-
To become part of the software architecture.
-
Means and techniques for continuous system monitoring and self-monitoring.
-
1.3.6.7 Major Challenge 7: Hardware virtualization for efficient SW engineering
Hardware virtualisation provides efficient abstraction from the physical hardware, thus allowing to decouple software engineering lifecycles from the underlying hardware. This is usually implemented either by full-scale hypervisors like VMware and KVM, or via containerisation as combination with the hosting operating system, e.g. Docker and Kubernetes. It enhances efficiency through resource isolation and allocation, and eases deployment by offering lightweight, portable, and scalable software packaging. Additionally, hardware-assisted virtualisation extensions such as Intel VT-x and AMD-V have improved performance and security by offloading virtualisation tasks to the hardware.
Hardware virtualisation is expected to offer numerous benefits, including increased flexibility, portability, and security for safety-critical and non-safety-critical applications.
-
Standardized Abstraction Models for hardware components to enable cross-platform compatibility, fostering ease of development and integration.
-
Timing Models: Developing reliable timing models to predict and verify real-time behaviour accurately.
-
Unified APIs for different hardware components to promote interoperability.
-
Performant run-time environments supporting shared memory access control, leveraging hypervisor technologies like XEN and KVM, as well as Bytecode/WebAssembly technologies.
-
Virtualisation of communication (Virtual Networks) supporting Quality of Service (QoS) mechanisms to guarantee real-time communication in mixed-criticality environments.
-
Hardware Abstraction for Sensors and Actuators, to achieve their interchangeability.
To realize the potential of hardware virtualisation, research and development will focus on three aspects.
-
Standard development methods and frameworks for the development of hardware abstractions, integrated with existing tools.
-
Verification and validation frameworks, supported by automation, which allow for the validation of applications within virtualisation, as well as the validation of specific target systems, to confirm performance and timeliness.
-
Run-time environments for safety-critical applications.
To cover these aspects, it is necessary to understand what they aim to achieve in software system engineering terms. To facilitate software development in virtual environments, the most common requirement in industry is to enable functional testing on virtual hardware. Such testing must provide fast execution feedback suitable for continuous engineering. Currently, there already exist techniques that try to achieve this30. What we also need is higher integration of such virtual platforms in common engineering development methodologies (e.g., Agile). To achieve other goals from the list above (e.g., performance and temporal properties evaluation), it is necessary to have more complex, detailed models of virtual hardware. Examples of such models currently exist31, but are extremely slow to execute. Besides integration of virtual platforms into existing methodologies and making execution of these models faster, it is necessary to observe the challenge around this effort in a wider scope. Development of virtual prototypes is not easy and often requires significant effort and investment. Moreover, management of platforms on which these prototypes execute is also a complex task (e.g. using BlueChi32). Finally, management of models, their variations, and integration activities with software repositories and test suites is challenging and time consuming. Therefore, we need approaches that facilitate faster development of virtual platforms, infrastructure around using them in software engineering, and management of both the models and the infrastructure. With these efforts, we hope to migrate a larger part of the development of embedded software systems to the cloud, facilitating collaboration and enabling higher engineering efficiency.
The following table illustrates the roadmaps for Embedded Software and Beyond. The assumption is that on the topics listed, that technology should be ready (TRL 8–9) in the respective time-frames.
MAJOR CHALLENGE | TOPIC | SHORT TERM (2025-2029) | MEDIUM TERM (2030-2034) | LONG TERM (2035 and beyond) |
---|---|---|---|---|
Major Challenge 1: Efficient engineering of embedded software |
Topic 1.1: Modelling-based software engineering | Model-based software engineering enabling systems to become part of SoS | Model inference to enable re-use of existing subsystems in SoS | Model-based testing taking re-use of uncontrolled SoS into account |
Topic 1.2 Digital twinning | Virtualisation of legacy systems | support virtual integration testing across variants | Support/allow (re)certification of systems via digital twins | |
Topic 1.3: Constraint environments |
Resource planning and scheduling Design for software evolution over time |
Embedded software architectures to enable SoS | Exploiting hybrid computer platforms, including efficient software portability | |
Topic 1.4: Software technology |
Virtualisation as tool for efficient engineering Technology for safe and dependable software ecosystems |
Interface management enabling systems to become part of SoS |
Develop new software architectures for edge computing Artificial intelligence to assist and support efforts in software engineering |
|
Topic 1.5: Software engineering tools | Co-simulation platforms | Middleware controlling dynamically embedded (mobile) hardware solutions Compilers and link to new hardware | Programming languages for developing large-scale applications for embedded SoS | |
Major Challenge 2: Continuous integration and deployment |
Topic 2.1: Continuous integration |
DevOps modelling Virtualisation |
Simulation on a virtual platform |
Digital twin Model-based engineering based on digital twins |
Topic 2.2: Verification and validation | Virtualisation of test platform | Model-based testing | Integration & orchestration platforms for IoT and SoS | |
Major Challenge 3: Life-cycle management of embedded software |
Topic 3.1: Rejuvenation of existing systems |
Software legacy and software rejuvenation Design for rejuvenating systems in a later phase |
End-of-life and evolving off-the-shelve/open- source solutions |
The cloud-for-edge continuum - “Write once, run anywhere” on this computing continuum Composability, properties contracts and orchestration systems Interoperability: must be ensured in integration platforms |
Topic 3.2: Managing complexity over time | Diagnostics of systems in the field | Continuous certification | Interplay between legacy | |
Topic 3.3: Managing Configurations over time | Full life-cycle configuration tacking | Methods and tools managing composability and system orchestration | Individualized systems configuration management | |
Topic 3.4: Evolvability of embedded software | Adaptable embedded software | Dynamical embedded software |
Autonomous embedded software Autonomous processes (IoT & edge embedded HW/SW co-design) |
|
Major Challenge 4: Embedding data analytics and AI |
Topic 4.1: Federated learning | Create federated learning at the edge in heterogeneous distributed systems | Federated intelligence at the edge |
Safe, trustworthy & explainable AI AI is playing several key roles in innovation, e.g. as a tool for SW development/ engineering Embedded intelligence |
Topic 4.2 Embedded Intelligence | Self-reflection: software AI framework supports acting on own system state | OS support for new HW (GPU, ASIC, neuromorphic computing,…) and platforms (Edge-AI,..) | Support dynamic adaption of systems | |
Topic 4.3: Data streaming in constraint environments | Feed streaming data into low-latency analysis and knowledge generation | Support processing by new HW (GPU, ASIC,…) | ||
Topic 4.4: Embedding AI accelerators | Accelerators and hardware/software co-design to speed up analysis and learning | Actual usage- based learning applied for accelerators and hardware/software co-design | Use of AI in autonomous systems | |
Major Challenge 5: Support for sustainability by embedded software |
Topic 5.1: resource-aware software engineering | Integration of green-aware aspects in software integration | Adaptive processing based on energy-awareness | |
Topic 5.2: Tools for energy efficient SW design | Rejuvenation technologies | Design for extending lifetime | Digital twins that support green deal and enable sustainability (e.g. contain power models) | |
Topic 5.3: Energy aware frameworks & libraries | Support monitoring/ reporting energy production / energy profiles | Support scale-able processing depending on available energy | Energy-optimal distributed computation | |
Topic 5.4: Management of computation power on embedded HW | SW/HW support for energy awareness of embedded systems | Support for embedded HPC | ||
Topic 5.5: Composable efficient abstraction | Enabling technologies for the second life of (legacy) cyber-physical systems | Establish relationships between power consumption and other quality properties | ||
Major Challenge 6: Software reliability and trust |
Topic 6.1: Reliability of software and new hardware |
Code coverage of reliability tooling and porting Simulation- and mock-up- based approaches for handling concurrency |
Embed reliability on software architecture level |
Use of quantum computing IoT digital twin simulation Validation and verification through simulation- and mock-up- based approaches for handling concurrency |
Topic 6.2: Robustness (trustworthy, secure, safe, privacy- aware) |
Trustworthy, secure, safe, privacy-aware Testing self-adapting systems using simulation |
Define a maturity model for robustness of embedded software and beyond | ||
Topic 6.3: Security and privacy as a service | Design for security and privacy as a service | Architecture for security and privacy as a service | ||
Major Challenge 7: Hardware virtualization for efficient SW engineering | Topic 7.1: development methods and frameworks for hardware abstractions | Modular building blocks available for creating abstraction layers for common multicore-CPU/SoC platforms | Design automation for abstraction layers built from formal HW description | Methodology and tools automating abstraction layer design, providing certain guarantees (safety, security, determinism etc.) |
Topic 7.2: validation of application frameworks | Support virtualized V&V of applications on abstraction layers for wide range of target systems and variants thereof | Automated validation of properties like safety, security and runtime determinism | ||
Topic 7.3: Run-time environments for safety-critical applications | Highly performant & analyse-able run-time environments supporting shared memory access control | Hardware abstraction frameworks fit to be certified for safety-critical domains (automotive, aeronautics,…) | Hardware abstraction frameworks fit for certification in highly safety-critical applications (e.g. ASIL-D in automotive) |